Development of Oral Cancer Risk Assessment Tool: An Exploratory Study
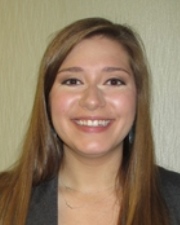
University of Minnesota
Krista J. Koehler, Neel A. Shimpi, Amit Acharya, Harshad Hedge, Gary Pack
Institute for Oral and Systemic Health, Biomedical Informatics Research Center
Research area: Dental Informatics
Background: Currently, there is no standard procedure for identifying patient risk of oral cancer. A combination of several etiological factors such as smoking, alcohol, oral Human Papilloma Virus and others have been associated with the risk of oral cancer. With advanced studies showing multiple etiological factors with synergistic effects acting on oral cancer development, it becomes difficult for health care providers to determine patient risk of oral cancer. We explored the design of an oral cancer risk assessment tool (OCRAT) based on MATLAB Artificial Neural Network (ANN).
Methods: Retrospective data was collected from the Marshfield Clinic data warehouse from 01/01/1979 to 06/06/2014. Structured data was filtered by applying inclusion and exclusion criteria to develop data sets of 300 cases and 300 controls, which were trained, tested, and validated using MATLAB. Only White, Non-Hispanic patients were included. The ANN had a total of 7 input neurons that included patient age, gender, white lesions, red lesions, submucous fibrosis, current smoker former smoker, 4 hidden nodes, and 2 outputs of either high or low risk of developing oral cancer. Performance function of the OCRAT prototype was analyzed on the validation set using a confusion matrix and mean square error (MSE).
Results: The validation set demonstrated a high specificity of 95.6%, sensitivity of 100%, while precision and recall were 95.2% and 100% respectively. The best epoch was at 11 with MSE of 0.09. Overall accuracy of MATLAB performance was 97.7%.
Conclusion: Progress designing a prototypical tool that had reasonable accuracy was made and provided an opportunity to understand the use of MATLAB and ANN methodology for the purpose of risk prediction. Further data mining through clinical notes may provide more information for input nodes including other known risk factors for oral cancer including alcohol consumption, thereby increasing the efficacy of OCRAT prototype.